Reinforcement Learning for Traffic Signal Control
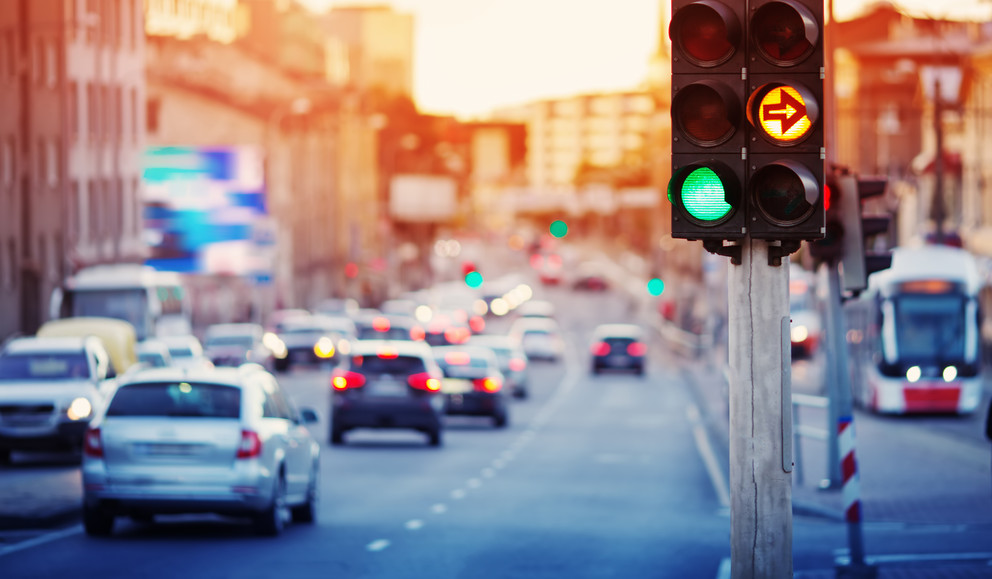
Project website
Key References:- AAAI'20 Toward A Thousand Lights: Decentralized Deep Reinforcement Learning for Large-Scale Traffic Signal Control
- AAAI'20 MetaLight: Value-based Meta-reinforcement Learning for Online Universal Traffic Signal Control
- CIKM'19 Learning Phase Competition for Traffic Signal Control
- CIKM'19 CoLight: Learning Network-level Cooperation for Traffic Signal Control
- CIKM'19 Learning Traffic Signal Control from Demonstrations
- KDD'19 PressLight: Learning Max Pressure Control to Coordinate Traffic Signals in Arterial Network
- WWW'19 demo CityFlow: A Multi-Agent Reinforcement Learning Environment for Large Scale City Traffic Scenario
- KDD'18 IntelliLight: A Reinforcement Learning Approach for Intelligent Traffic Light Control
- Survey Survey on traffic signal control
Deep Learning for Spatial-Temporal Prediction
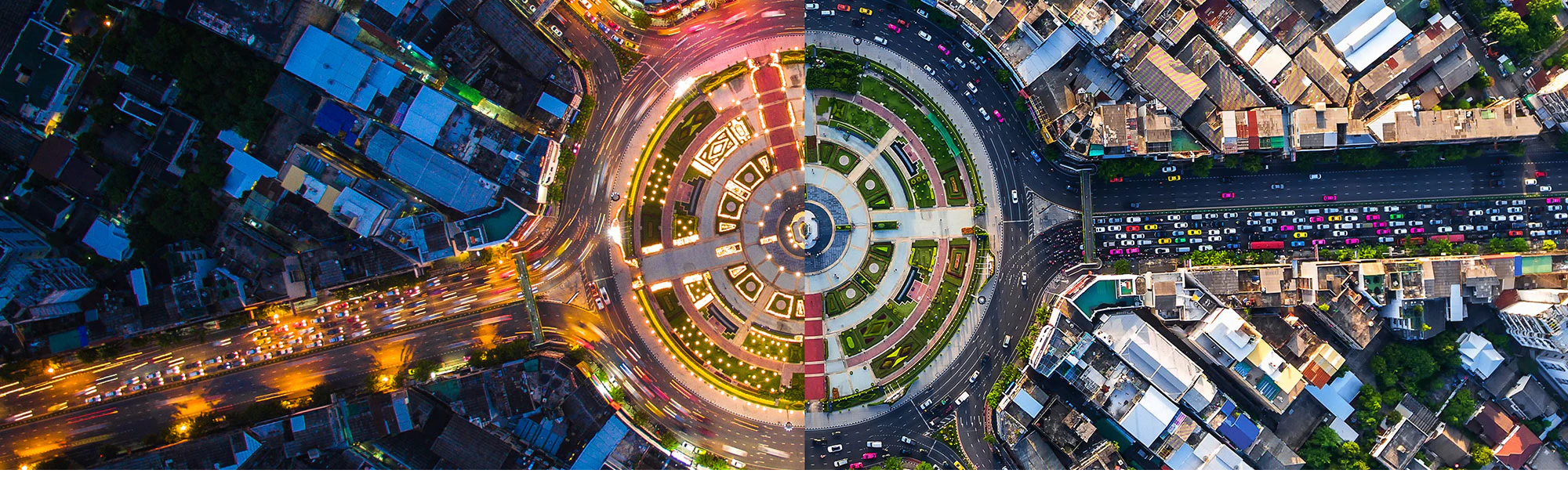
Project website
Key References:- WWW'19 Learning from Multiple Cities: A Meta-Learning Approach to Spatial-Temporal Prediction
- AAAI'19 Revisiting Spatial-Temporal Similarity: A Deep Learning Framework for Traffic Prediction
- AAAI'18 Deep Multi-View Spatial-Temporal Network for Taxi Demand Prediction
Urban Data Computing
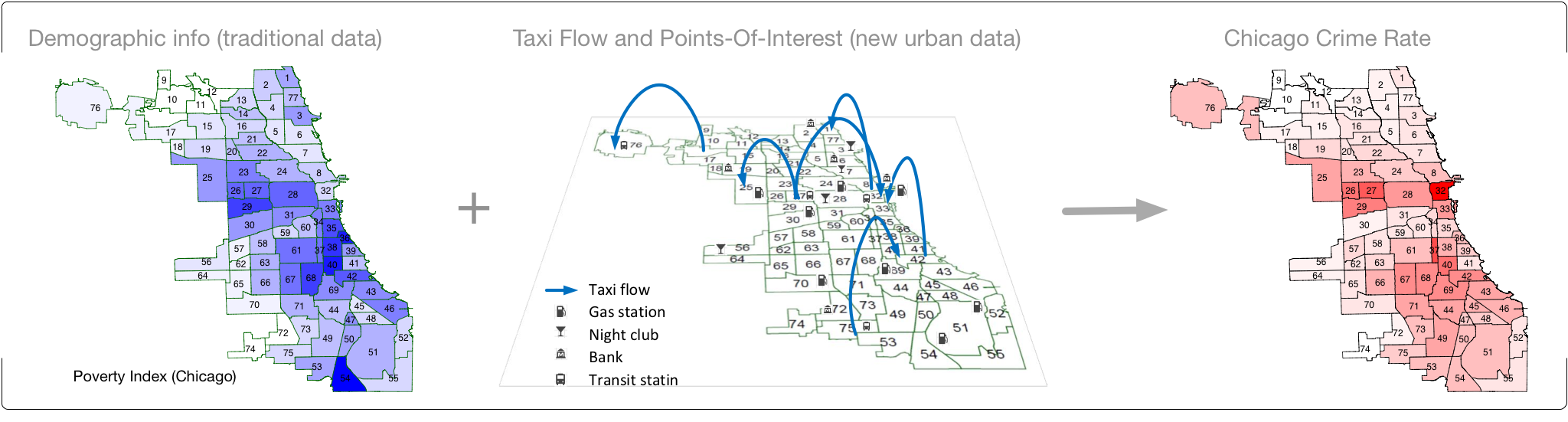
Increasing amount of urban data are being accumulated in the digital form, such as human trace traffic, venues, crime, weather, local events, vehicle collisions, and many more. Many cities in U.S. (e.g., New York City, Chicago, and Los Angeles) have joined the open data initiative and created websites to release the city data to the public. Analyzing such data could empower us to address many critical urban issues such as crime, traffic jam, education, health, and life quality. Our recent study has shown that using taxi flow data and Point-Of-Interest data can significantly improve crime rate inference in Chicago (KDD'16).
Funding: This research project is funded by NSF CAREER award (2017-2022). The crime data analysis research is funded by NSF award #1544455 (2015-2017).
-
Key References:
- CIKM'19 Unsupervised Representation Learning of Spatial Data via Multimodal Embedding
- WWW'19 Learning Task-Specific City Region Partitions
- IMWUT'18 Inferring Mobility Relationship via Graph Embedding
- TBD'17 Non-Stationary Model for Crime Rate Inference Using Modern Urban Data
- CIKM'17 Region Representation Learning via Mobility Flow
- KDD'16 Crime Rate Inference with Big Data
Trajectory Analysis
The advances in location-acquisition technologies and the prevalence of location-based services have generated massive spatial trajectory data, which represent the mobility of a diversity of moving objects, such as people, vehicles, and animals. Such trajectories offer us unprecedented information to understand moving objects and locations that could benefit a broad range of applications in business, transportation, ecology, and many more.Collaborators: Roland Kays (NC Museum of Natural Sciences and Department of Forestry & Environmental Resources, North Carolina State University), Margaret Crofoot (Anthropology Department, UC Davis), Wang-Chien Lee (Computer Science and Engineering, Penn State), Stephen Matthews (Department of Sociology and Criminology, Penn State)
Trajectory Analysis: Semantic Trajectory Mining with Contexts
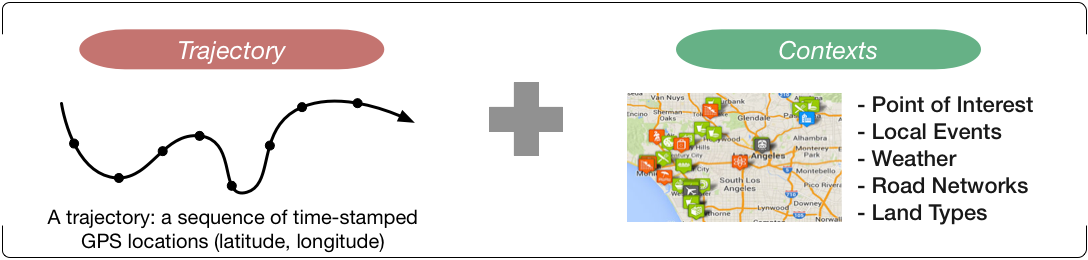
Funding: This research is funded by NSF award #1618448 (2016-2019).
-
Key References:
- CIKM'16 Where Did You Go: Personalized Annotation of Mobility Records
- WWW'15 Semantic Annotation of Mobility Data using Social Media
Trajectory Analysis: Inferring Social Relationships based on Spatial-Temporal Interactions
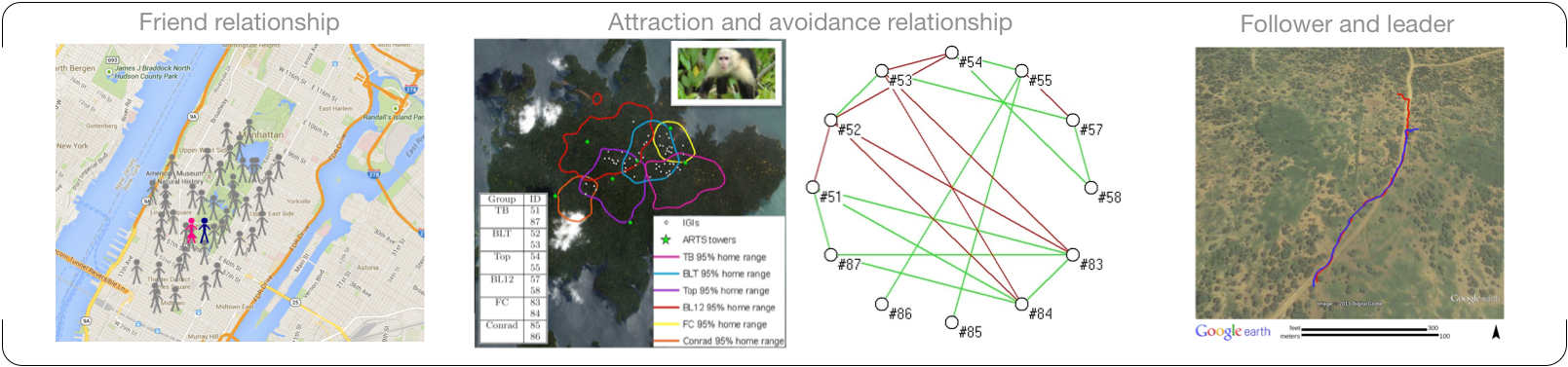
Spatiotemporal data collected from GPS has the potential to provide insights into the relationship dynamics of individuals. For example, people gathering on a Saturday night could be an informative signal for a friend relationship, while being together during the day on weekdays indicates a potential colleague relationship. Our recent research projects have studied attraction/avoidance relationship (VLDB'14), friend relationship (ICDM'14, SSTD'11), follower/leader relationship (ICDM'13), and moving object clusters (VLDB'10).
-
Key References:
- VLDB'14 Attraction and Avoidance Detection from Movements
- ICDM'14 PGT: Measuring Mobility Relationship Using Personal, Global and Temporal Factors
- ICDM'13 Mining Following Relationships in Movement Data
- SSTD'11 Mining Significant Time Intervals for Relationship Detection
- VLDB'10 Swarm: Mining Relaxed Temporal Moving Object Clusters
Trajectory Analysis: Periodic Behaviour Analysis
We have designed algorithm, Periodica (KDD'10), to mine multiple interleaved periodic behaviors in complex movement. This is the first work that studies how to automatically detect the hidden periods in the movement. Periodicity can be successfully used to fill in missing data and predict future movement (DAMI'12).
Due to the limitations of positioning technology and data collection mechanisms, movement data collected from GPS or sensors could be highly sparse, noisy and unsynchronized. In our recent work (KDD'12, TKDE'15), a segment-and-overlay idea is explored to uncover the hidden period: Even when the observations are incomplete, the limited periodic observations will be clustered together if data is overlaid with the correct period.
-
Key References:
- TKDE'15 ePeriodicity: Mining Event Periodicity from Incomplete Observations
- KDD'12 Mining Periodicity for Sparse and Incomplete Event Data
- DAMI'12 Mining Periodic Behaviors of Object Movements for Animal and Biological Sustainability Studies
- KDD'10 Mining Periodic Behaviors for Moving Objects
Environmental Data Mining
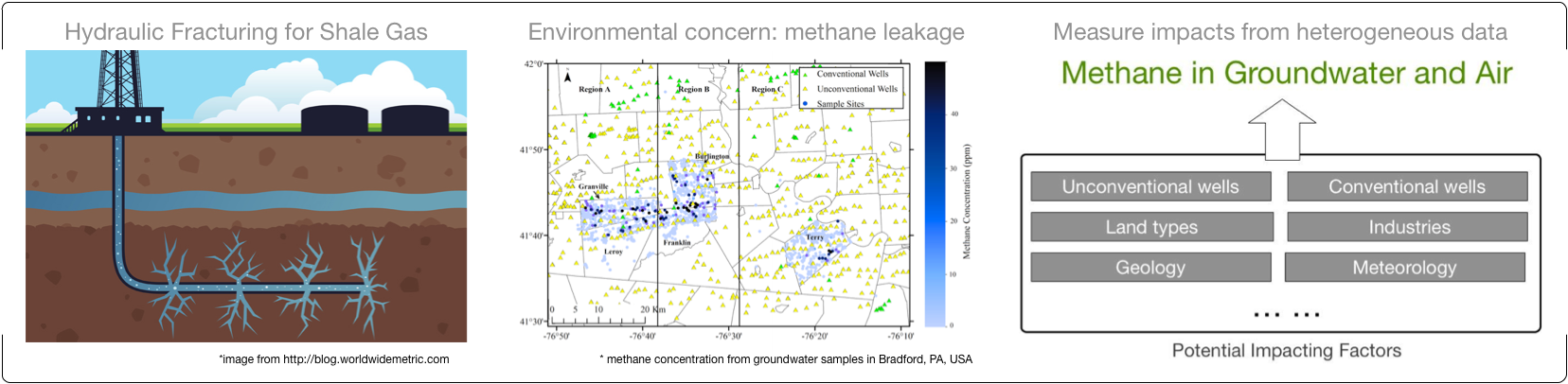
High volume hydraulic fracturing, also called fracking, allows drillers to extract natural gas from shale deep within the earth. But such natural gas development also has led to environmental concerns. Methane gas sometimes escapes from shale gas wells and can contaminate water resources or leak into the atmosphere where it contributes to greenhouse gas emissions. We explore how to analyze the heterogeneous spatial data that describe distributions of methane concentrations in natural waters.
Funding: This research is funded by NSF award #1639150 (2016-2019) and CCRINGSS center at Penn State (2015-2016).
Collaborators: Susan Brantley (Department of Geosciences, Penn State)
-
Key References:
- SDM'17 Discovery of Causal Time Intervals
- A data-driven approach to evaluate environmental impacts of shale-gas drilling, talk given at Shale Network Workshop, 2016
- Statistical Analysis and Data Mining on Water Quality Data, talk given at Shale Network Workshop, 2015 .